Prediction
One of the important problems in climate research is a skillful prediction of the occurrence of an El Niño event, which is a strong increase in the sea surface temperature in the eastern Pacific Ocean, for lead times longer than six months. Such predictions are needed for taking adequate measures (e.g. by farmers) to mitigate the effects of El Niño.
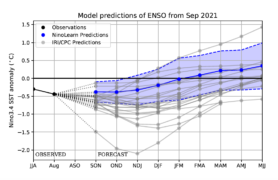
Model predictions of ENSO from September 2021
In the figures, the ONI prediction and its uncertainty (blue) are shown from a starting month (displayed at the top of the figure) and compared with predictions (grey) from the Climate Prediction Center (CPC) and the Institute Research Institute for Climate and Society (IRI). These figures will be updated three months.
What’s new?
At the Centre for Complex Systems Studies (CCSS), we developed a machine learning based prediction system for El Niño which predicts not only the main index of El Niño, the Ocean Nino Index (ONI), but also its uncertainty for lead times up to nine months. We use a Gaussian Density Neural Network (GDNN) which is trained using a limited features set, consisting of data from several observations relevant to El Niño, such as the Warm Water Volume and the Western Pacific wind-stress anomalies. A detailed description of the GDNN methodology can be found in Petersik and Dijkstra (2020) and an overview of the use of machine learning techniques in El Niño prediction in Dijkstra et al. (2019).
References:
Dijkstra, H. A., Petersik, P., Hernández-García, E., & López, C., 2019: The application of machine learning techniques to improve El Niño prediction skill. Frontiers of Physics, 7, 1–13.
Petersik, P. J. and Dijkstra, H. A., 2020: Probabilistic forecasting of El Niño using neural network models. Geophysical Research Letters, 47. , e2019GLO86423.
Previous predictions:
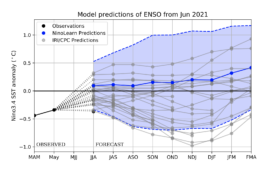
Model predictions of ENSO from June 2021